Data Science at Home
Episodes
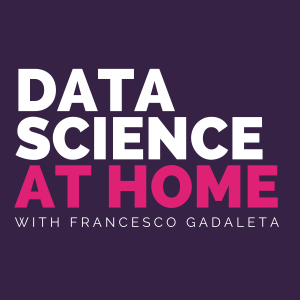
Tuesday Nov 12, 2019
Tuesday Nov 12, 2019
What happens to a neural network trained with random data?
Are massive neural networks just lookup tables or do they truly learn something?
Today’s episode will be about memorisation and generalisation in deep learning, with Stanislaw Jastrzębski from New York University.
Stan spent two summers as a visiting student with Prof. Yoshua Bengio and has been working on
Understanding and improving how deep network generalise
Representation Learning
Natural Language Processing
Computer Aided Drug Design
What makes deep learning unique?
I have asked him a few questions for which I was looking for an answer for a long time. For instance, what is deep learning bringing to the table that other methods don’t or are not capable of? Stan believe that the one thing that makes deep learning special is representation learning. All the other competing methods, be it kernel machines, or random forests, do not have this capability. Moreover, optimisation (SGD) lies at the heart of representation learning in the sense that it allows finding good representations.
What really improves the training quality of a neural network?
We discussed about the accuracy of neural networks depending pretty much on how good the Stochastic Gradient Descent method is at finding minima of the loss function. What would influence such minima?Stan's answer has revealed that training set accuracy or loss value is not that interesting actually. It is relatively easy to overfit data (i.e. achieve the lowest loss possible), provided a large enough network, and a large enough computational budget. However, shape of the minima, or performance on validation sets are in a quite fascinating way influenced by optimisation. Optimisation in the beginning of the trajectory, steers such trajectory towards minima of certain properties that go much further than just training accuracy.
As always we spoke about the future of AI and the role deep learning will play.
I hope you enjoy the show!
Don't forget to join the conversation on our new Discord channel. See you there!
References
Homepage of Stanisław Jastrzębski https://kudkudak.github.io/
A Closer Look at Memorization in Deep Networks https://arxiv.org/abs/1706.05394
Three Factors Influencing Minima in SGD https://arxiv.org/abs/1711.04623
Don't Decay the Learning Rate, Increase the Batch Size https://arxiv.org/abs/1711.00489
Stiffness: A New Perspective on Generalization in Neural Networks https://arxiv.org/abs/1901.09491
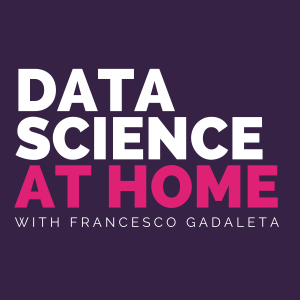
Tuesday Nov 05, 2019
Deeplearning is easier when it is illustrated (with Jon Krohn) (Ep. 86)
Tuesday Nov 05, 2019
Tuesday Nov 05, 2019
In this episode I speak with Jon Krohn, author of Deeplearning Illustrated a book that makes deep learning easier to grasp.
We also talk about some important guidelines to take into account whenever you implement a deep learning model, how to deal with bias in machine learning used to match jobs to candidates and the future of AI.
You can purchase the book from informit.com/dsathome with code DSATHOME and get 40% off books/eBooks and 60% off video training
![[RB] Replicating GPT-2, the most dangerous NLP model (with Aaron Gokaslan) (Ep. 83)](https://pbcdn1.podbean.com/imglogo/ep-logo/pbblog1799802/data_science_at_home_podcast_cover_300x300.png)
Friday Oct 18, 2019
Friday Oct 18, 2019
Join the discussion on our Discord server
In this episode, I am with Aaron Gokaslan, computer vision researcher, AI Resident at Facebook AI Research. Aaron is the author of OpenGPT-2, a parallel NLP model to the most discussed version that OpenAI decided not to release because too accurate to be published.
We discuss about image-to-image translation, the dangers of the GPT-2 model and the future of AI. Moreover, Aaron provides some very interesting links and demos that will blow your mind!
Enjoy the show!
References
Multimodal image to image translation (not all mentioned in the podcast but recommended by Aaron)
Pix2Pix:
https://phillipi.github.io/pix2pix/
CycleGAN:
https://junyanz.github.io/CycleGAN/
GANimorph
Paper: https://arxiv.org/abs/1808.04325
Code: https://github.com/brownvc/ganimorph
UNIT:https://arxiv.org/abs/1703.00848
MUNIT:https://github.com/NVlabs/MUNIT
DRIT: https://github.com/HsinYingLee/DRIT
GPT-2 and related
Try OpenAI's GPT-2: https://talktotransformer.com/
Blogpost: https://blog.usejournal.com/opengpt-2-we-replicated-gpt-2-because-you-can-too-45e34e6d36dc
The Original Transformer Paper: https://arxiv.org/abs/1706.03762
Grover: The FakeNews generator and detector: https://rowanzellers.com/grover/
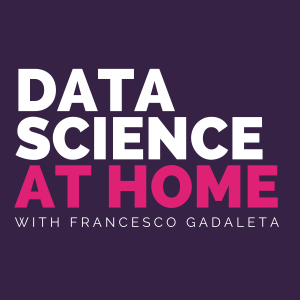
Tuesday Oct 15, 2019
What is wrong with reinforcement learning? (Ep. 82)
Tuesday Oct 15, 2019
Tuesday Oct 15, 2019
Join the discussion on our Discord server
After reinforcement learning agents doing great at playing Atari video games, Alpha Go, doing financial trading, dealing with language modeling, let me tell you the real story here.In this episode I want to shine some light on reinforcement learning (RL) and the limitations that every practitioner should consider before taking certain directions. RL seems to work so well! What is wrong with it?
Are you a listener of Data Science at Home podcast? A reader of the Amethix Blog? Or did you subscribe to the Artificial Intelligence at your fingertips newsletter? In any case let’s stay in touch! https://amethix.com/survey/
References
Emergence of Locomotion Behaviours in Rich Environments https://arxiv.org/abs/1707.02286
Rainbow: Combining Improvements in Deep Reinforcement Learning https://arxiv.org/abs/1710.02298
AlphaGo Zero: Starting from scratch https://deepmind.com/blog/article/alphago-zero-starting-scratch
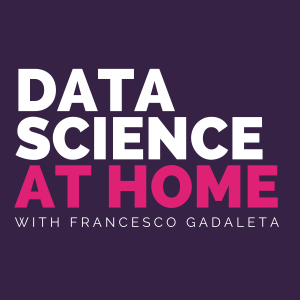
Monday Sep 23, 2019
Monday Sep 23, 2019
Join the discussion on our Discord server
In this episode, I am with Aaron Gokaslan, computer vision researcher, AI Resident at Facebook AI Research. Aaron is the author of OpenGPT-2, a parallel NLP model to the most discussed version that OpenAI decided not to release because too accurate to be published.
We discuss about image-to-image translation, the dangers of the GPT-2 model and the future of AI. Moreover, Aaron provides some very interesting links and demos that will blow your mind!
Enjoy the show!
References
Multimodal image to image translation (not all mentioned in the podcast but recommended by Aaron)
Pix2Pix:
https://phillipi.github.io/pix2pix/
CycleGAN:
https://junyanz.github.io/CycleGAN/
GANimorph
Paper: https://arxiv.org/abs/1808.04325
Code: https://github.com/brownvc/ganimorph
UNIT:https://arxiv.org/abs/1703.00848
MUNIT:https://github.com/NVlabs/MUNIT
DRIT: https://github.com/HsinYingLee/DRIT
GPT-2 and related
Try OpenAI's GPT-2: https://talktotransformer.com/
Blogpost: https://blog.usejournal.com/opengpt-2-we-replicated-gpt-2-because-you-can-too-45e34e6d36dc
The Original Transformer Paper: https://arxiv.org/abs/1706.03762
Grover: The FakeNews generator and detector: https://rowanzellers.com/grover/
![[RB] Complex video analysis made easy with Videoflow (Ep. 75)](https://pbcdn1.podbean.com/imglogo/ep-logo/pbblog1799802/data_science_at_home_podcast_cover_300x300.png)
Thursday Aug 29, 2019
[RB] Complex video analysis made easy with Videoflow (Ep. 75)
Thursday Aug 29, 2019
Thursday Aug 29, 2019
In this episode I am with Jadiel de Armas, senior software engineer at Disney and author of Videflow, a Python framework that facilitates the quick development of complex video analysis applications and other series-processing based applications in a multiprocessing environment.
I have inspected the videoflow repo on Github and some of the capabilities of this framework and I must say that it’s really interesting. Jadiel is going to tell us a lot more than what you can read from Github
References
Videflow Github official repository https://github.com/videoflow/videoflow
![[RB] Validate neural networks without data with Dr. Charles Martin (Ep. 74)](https://pbcdn1.podbean.com/imglogo/ep-logo/pbblog1799802/data_science_at_home_podcast_cover_300x300.png)
Tuesday Aug 27, 2019
[RB] Validate neural networks without data with Dr. Charles Martin (Ep. 74)
Tuesday Aug 27, 2019
Tuesday Aug 27, 2019
In this episode, I am with Dr. Charles Martin from Calculation Consulting a machine learning and data science consulting company based in San Francisco. We speak about the nuts and bolts of deep neural networks and some impressive findings about the way they work.
The questions that Charles answers in the show are essentially two:
Why is regularisation in deep learning seemingly quite different than regularisation in other areas on ML?
How can we dominate DNN in a theoretically principled way?
References
The WeightWatcher tool for predicting the accuracy of Deep Neural Networks https://github.com/CalculatedContent/WeightWatcher
Slack channel https://weightwatcherai.slack.com/
Dr. Charles Martin Blog http://calculatedcontent.com and channel https://www.youtube.com/c/calculationconsulting
Implicit Self-Regularization in Deep Neural Networks: Evidence from Random Matrix Theory and Implications for Learning - Charles H. Martin, Michael W. Mahoney
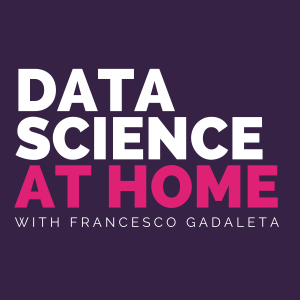
Wednesday Aug 21, 2019
How to cluster tabular data with Markov Clustering (Ep. 73)
Wednesday Aug 21, 2019
Wednesday Aug 21, 2019
In this episode I explain how a community detection algorithm known as Markov clustering can be constructed by combining simple concepts like random walks, graphs, similarity matrix. Moreover, I highlight how one can build a similarity graph and then run a community detection algorithm on such graph to find clusters in tabular data.
You can find a simple hands-on code snippet to play with on the Amethix Blog
Enjoy the show!
References
[1] S. Fortunato, “Community detection in graphs”, Physics Reports, volume 486, issues 3-5, pages 75-174, February 2010.
[2] Z. Yang, et al., “A Comparative Analysis of Community Detection Algorithms on Artificial Networks”, Scientific Reports volume 6, Article number: 30750 (2016)
[3] S. Dongen, “A cluster algorithm for graphs”, Technical Report, CWI (Centre for Mathematics and Computer Science) Amsterdam, The Netherlands, 2000.
[4] A. J. Enright, et al., “An efficient algorithm for large-scale detection of protein families”, Nucleic Acids Research, volume 30, issue 7, pages 1575-1584, 2002.
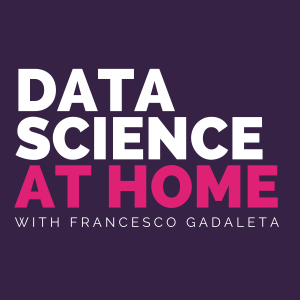
Tuesday Aug 06, 2019
Training neural networks faster without GPU (Ep. 71)
Tuesday Aug 06, 2019
Tuesday Aug 06, 2019
Training neural networks faster usually involves the usage of powerful GPUs. In this episode I explain an interesting method from a group of researchers from Google Brain, who can train neural networks faster by squeezing the hardware to their needs and making the training pipeline more dense.
Enjoy the show!
References
Faster Neural Network Training with Data Echoinghttps://arxiv.org/abs/1907.05550
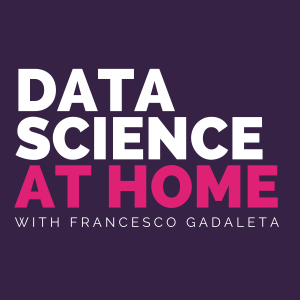
Tuesday Jul 23, 2019
Validate neural networks without data with Dr. Charles Martin (Ep. 70)
Tuesday Jul 23, 2019
Tuesday Jul 23, 2019
In this episode, I am with Dr. Charles Martin from Calculation Consulting a machine learning and data science consulting company based in San Francisco. We speak about the nuts and bolts of deep neural networks and some impressive findings about the way they work.
The questions that Charles answers in the show are essentially two:
Why is regularisation in deep learning seemingly quite different than regularisation in other areas on ML?
How can we dominate DNN in a theoretically principled way?
References
The WeightWatcher tool for predicting the accuracy of Deep Neural Networks https://github.com/CalculatedContent/WeightWatcher
Slack channel https://weightwatcherai.slack.com/
Dr. Charles Martin Blog http://calculatedcontent.com and channel https://www.youtube.com/c/calculationconsulting
Implicit Self-Regularization in Deep Neural Networks: Evidence from Random Matrix Theory and Implications for Learning - Charles H. Martin, Michael W. Mahoney