Data Science at Home
Episodes
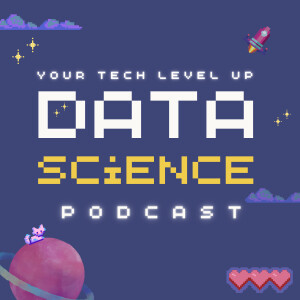
Wednesday May 07, 2025
Wednesday May 07, 2025
Welcome to DSH/Warcoded
We explore how AI is transforming ISR (Intelligence, Surveillance, Reconnaissance)—from satellite imagery to drone feeds. In this episode:
🔍 Computer vision for target ID📡 Predictive surveillance & pattern-of-life modeling🧠 LLMs for SIGINT & OSINT intelligence briefings🌍 Real-world examples: Ukraine, Gaza & more
Listen now and see how machines are learning to see, predict, and inform at the edge of modern conflict.
Sponsors
Warcoded is proudly sponsored by Amethix Technologies. At the intersection of ethics and engineering, Amethix creates AI systems that don’t just function—they adapt, learn, and serve. With a focus on dual-use innovation, Amethix is shaping a future where intelligent machines extend human capability, not replace it. Discover more at amethix.com
Warcoded is brought to you by Intrepid AI. From drones to satellites, Intrepid AI gives engineers and defense innovators the tools to prototype, simulate, and deploy autonomous systems with confidence. Whether it's in the sky, on the ground, or in orbit—if it's intelligent and mobile, Intrepid helps you build it. Learn more at intrepid.ai
#AI #defensetech #ISR #LLM #Warcoded #DataScienceAtHome #OSINT #SIGINT #dronewarfare
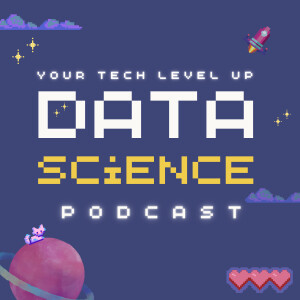
Monday Dec 23, 2024
Monday Dec 23, 2024
In this episode, we dive into the transformative world of AI, data analytics, and cloud infrastructure with Josh Miramant, CEO of Blue Orange Digital. As a seasoned entrepreneur with over $25 million raised across ventures and two successful exits, Josh shares invaluable insights on scaling data-driven businesses, integrating machine learning frameworks, and navigating the rapidly evolving landscape of cloud data architecture. From generative AI to large language models, Josh explores cutting-edge trends shaping financial services, real estate, and consumer goods.
Tune in for a masterclass in leveraging data for impact and innovation!
Links
https://blueorange.digital/
https://blueorange.digital/blog/a-data-intelligence-platform-what-is-it/
https://blueorange.digital/blog/ai-makes-bi-tools-accessible-to-anyone/
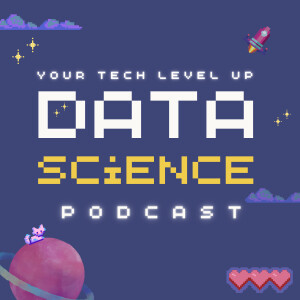
Monday Nov 25, 2024
Humans vs. Bots: Are You Talking to a Machine Right Now? (Ep. 273)
Monday Nov 25, 2024
Monday Nov 25, 2024
In this episode of Data Science at Home, host Francesco Gadaleta dives deep into the evolving world of AI-generated content detection with experts Souradip Chakraborty, Ph.D. grad student at the University of Maryland, and Amrit Singh Bedi, CS faculty at the University of Central Florida.
Together, they explore the growing importance of distinguishing human-written from AI-generated text, discussing real-world examples from social media to news. How reliable are current detection tools like DetectGPT? What are the ethical and technical challenges ahead as AI continues to advance? And is the balance between innovation and regulation tipping in the right direction?
Tune in for insights on the future of AI text detection and the broader implications for media, academia, and policy.
Chapters
00:00 - Intro
00:23 - Guests: Souradip Chakraborty and Amrit Singh Bedi
01:25 - Distinguish Text Generation By AI
04:33 - Research on Safety and Alignment of Generative Model
06:01 - Tools to Detect Generated AI Text
11:28 - Water Marking
18:27 - Challenges in Detecting Large Documents Generated by AI
23:34 - Number of Tokens
26:22 - Adversarial Attack
29:01 - True Positive and False Positive of Detectors
31:01 - Limit of Technologies
41:01 - Future of AI Detection Techniques
46:04 - Closing Thought
Subscribe to our new YouTube channel https://www.youtube.com/@DataScienceatHome
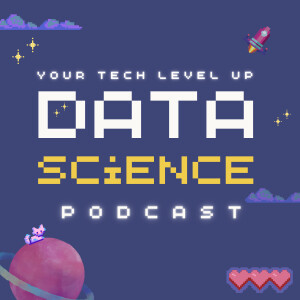
Saturday Oct 12, 2024
What Big Tech Isn’t Telling You About AI (Ep. 267)
Saturday Oct 12, 2024
Saturday Oct 12, 2024
Are AI giants really building trustworthy systems? A groundbreaking transparency report by Stanford, MIT, and Princeton says no. In this episode, we expose the shocking lack of transparency in AI development and how it impacts bias, safety, and trust in the technology. We’ll break down Gary Marcus’s demands for more openness and what consumers should know about the AI products shaping their lives.
Check our new YouTube channel https://www.youtube.com/@DataScienceatHome and Subscribe!
Cool links
https://mitpress.mit.edu/9780262551069/taming-silicon-valley/
http://garymarcus.com/index.html
![Kaggle Kommando’s Data Disco: Laughing our Way Through AI Trends (Ep. 265) [RB]](https://pbcdn1.podbean.com/imglogo/image-logo/1799802/dsh-cover-2_300x300.jpg)
Tuesday Oct 01, 2024
Kaggle Kommando’s Data Disco: Laughing our Way Through AI Trends (Ep. 265) [RB]
Tuesday Oct 01, 2024
Tuesday Oct 01, 2024
In this episode, join me and the Kaggle Grand Master, Konrad Banachewicz, for a hilarious journey into the zany world of data science trends. From algorithm acrobatics to AI, creativity, Hollywood movies, and music, we just can't get enough. It's the typical episode with a dose of nerdy comedy you didn't know you needed. Buckle up, it's a data disco, and we're breaking down the binary!
Sponsors
Intrepid AI is an AI assisted all-in-one platform for robotics teams. Build robotics applications in minutes, not months.
Learn what the new year holds for ransomware as a service, Active Directory, artificial intelligence and more when you download the 2024 Arctic Wolf Labs Predictions Report today at arcticwolf.com/datascience
🔗 Links Mentioned in the Episode:
Generative AI for time series: TimeGPT Documentation
Lag-llama: GitHub (Note: The benchmark results on this one are pretty horrible)
Open source LLM: Olmo Blog Post
Quantization for LLM: Hugging Face Guide
And finally, don't miss Konrad's Substack for more nerdy goodness! (If you're there already, be there again! 😄)
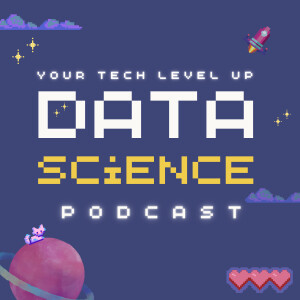
Monday Jul 22, 2024
Low-Code Magic: Can It Transform Analytics? (Ep. 260)
Monday Jul 22, 2024
Monday Jul 22, 2024
Join us as David Marom, Head of Panoply Business, explores the benefits of all-in-one data platforms. Learn how tech stack consolidation boosts efficiency, improves data accuracy, and cuts costs. David shares insights on overcoming common challenges, enhancing data governance, and success stories from organizations thriving with Panoply.
Sponsors
Arctic Wolf Learn what the new year holds for ransomware as a service, Active Directory, artificial intelligence and more when you download the 2024 Arctic Wolf Labs Predictions Report today at arcticwolf.com/datascience
Intrepid AI (https://intrepid.ai) is an AI assisted all-in-one platform for robotics teams. Build robotics applications in minutes, not months
References
Connect and analyze ALL of your data https://panoply.io/
https://blog.panoply.io/raw-data-to-dashboards-in-just-10-steps
https://blog.panoply.io/understanding-etl-extract-transform-and-load-data-to-boost-your-business-intelligence
Blog: The Transformative Power of an All-in-One Data Platform
Whitepaper: Eradicating Platform Inefficiencies
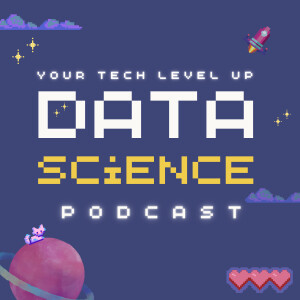
Saturday Jun 22, 2024
Do you really know how GPUs work? (Ep. 259)
Saturday Jun 22, 2024
Saturday Jun 22, 2024
Join us in this exciting episode of the Data Science at Home podcast. It's all about GPUs. We'll take you on a journey through the inner workings of these powerful processors, explaining how they handle complex computations and drive everything from gaming graphics to scientific simulations. Whether you're a budding programmer or a tech enthusiast, understanding GPUs is key to unlocking new levels of performance and efficiency in your projects. Tune in and get ready to turbocharge your tech knowledge!
Sponsors
Intrepid AI (https://intrepid.ai) is an AI assisted all-in-one platform for robotics teams. Build robotics applications in minutes, not months.
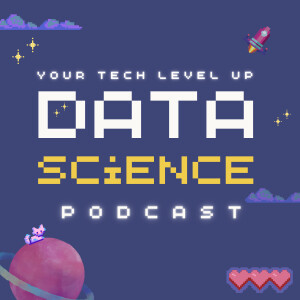
Thursday Mar 07, 2024
Kaggle Kommando's Data Disco: Laughing our Way Through AI Trends (Ep. 252)
Thursday Mar 07, 2024
Thursday Mar 07, 2024
In this episode, join me and the Kaggle Grand Master, Konrad Banachewicz, for a hilarious journey into the zany world of data science trends. From algorithm acrobatics to AI, creativity, Hollywood movies, and music, we just can't get enough. It's the typical episode with a dose of nerdy comedy you didn't know you needed. Buckle up, it's a data disco, and we're breaking down the binary!
Sponsors
Intrepid AI is an AI assisted all-in-one platform for robotics teams. Build robotics applications in minutes, not months.
Learn what the new year holds for ransomware as a service, Active Directory, artificial intelligence and more when you download the 2024 Arctic Wolf Labs Predictions Report today at arcticwolf.com/datascience
🔗 Links Mentioned in the Episode:
Generative AI for time series: TimeGPT Documentation
Lag-llama: GitHub (Note: The benchmark results on this one are pretty horrible)
Open source LLM: Olmo Blog Post
Quantization for LLM: Hugging Face Guide
And finally, don't miss Konrad's Substack for more nerdy goodness! (If you're there already, be there again! 😄)
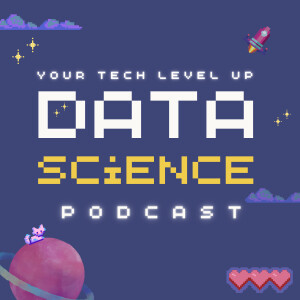
Tuesday Jan 30, 2024
Is SQream the fastest big data platform? (Ep. 250)
Tuesday Jan 30, 2024
Tuesday Jan 30, 2024
Join us in a dynamic conversation with Yori Lavi, Field CTO at SQream, as we unravel the data analytics landscape. From debunking the data lakehouse hype to SQream's GPU-based magic, discover how extreme data challenges are met with agility. Yori shares success stories, insights into SQream's petabyte-scale capabilities, and a roadmap to breaking down organizational bottlenecks in data science. Dive into the future of data analytics with SQream's commitment to innovation, leaving legacy formats behind and leading the charge in large-scale, cost-effective data projects. Tune in for a dose of GPU-powered revolution!
References
SQream - GPU-based Big Data Platform
Patents Assigned to SQREAM TECHNOLOGIES LTD
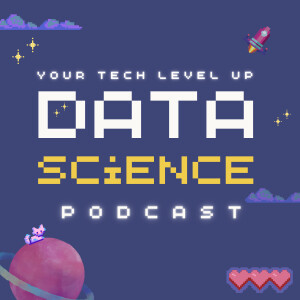
Monday Jan 08, 2024
Careers, Skills, and the Evolution of AI (Ep. 248)
Monday Jan 08, 2024
Monday Jan 08, 2024
!!WARNING!!
Due to some technical issues the volume is not always constant during the show. I sincerely apologise for any inconvenienceFrancesco
In this episode, I speak with Richie Cotton, Data Evangelist at DataCamp, as he delves into the dynamic intersection of AI and education. Richie, a seasoned expert in data science and the host of the podcast, brings together a wealth of knowledge and experience to explore the evolving landscape of AI careers, the skills essential for generative AI technologies, and the symbiosis of domain expertise and technical skills in the industry.
References
Become a generative AI developer in this FREE code-along series. Learn to build a chatbot using the OpenAI API, the Pinecone API, and LangChain, and learn to build NLP and image applications with Hugging Face. https://www.datacamp.com/ai-code-alongs
Learn to use ChatGPT and the OpenAI API in the OpenAI Fundamentals skill track. https://www.datacamp.com/tracks/openai-fundamentals
Get started with deep learning using PyTorch in the Introduction to Deep Learning with PyTorch course. https://www.datacamp.com/courses/introduction-to-deep-learning-with-pytorch