Data Science at Home
Episodes
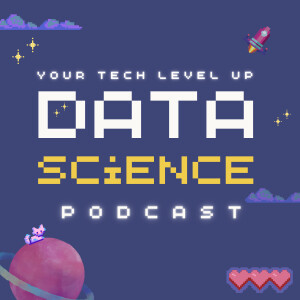
Wednesday Jun 18, 2025
Brains in the Machine: The Rise of Neuromorphic Computing (Ep. 285)
Wednesday Jun 18, 2025
Wednesday Jun 18, 2025
In this episode of Data Science at Home, we explore the fascinating world of neuromorphic computing — a brain-inspired approach to computation that could reshape the future of AI and robotics. The episode breaks down how neuromorphic systems differ from conventional AI architectures like transformers and LLMs, diving into spiking neural networks (SNNs), their benefits in energy efficiency and real-time processing, and their limitations in training and scalability. Real-world applications are highlighted, including low-power drones, hearing aids, and event-based cameras. Francesco closes with a vision of hybrid systems where neuromorphic chips and LLMs coexist, blending biological inspiration with modern AI.
📚 References
SpikingJelly: https://github.com/fangwei123456/spikingjelly
Norse: https://github.com/norse/norse
IBM TrueNorth: https://research.ibm.com/blog/brain-inspired-chip
Intel Loihi 2: https://www.intel.com/content/www/us/en/research/neuromorphic-computing.html
SpiNNaker: https://apt.cs.manchester.ac.uk/projects/SpiNNaker/
BioRobotics Institute: https://www.santannapisa.it/en/institute/biorobotics
🎙️ Sponsors
MillionaireMatch — The elite platform for high-achieving singles🌐 https://www.millionairematch.com
AGNTCY — The open source collective building the Internet of Agents🌐 https://www.agntcy.org
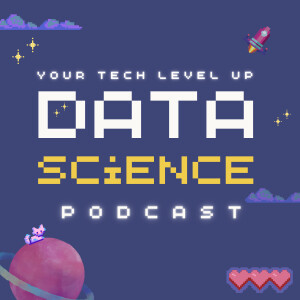
Wednesday May 14, 2025
Wednesday May 14, 2025
In this gripping follow-up, we dive into how AI is transforming kinetic operations—from identifying a threat to executing a strike.
🔍 Highlights from this episode:
How AI compresses the OODA loop (Observe, Orient, Decide, Act)
The spectrum of autonomy: human-on-the-loop vs. human-out-of-the-loop
Real-world systems like loitering munitions (Switchblade, Harpy) and Selective Ground Response AI (SGR-AI)
The ethical and legal dimensions of delegating lethal decisions to machines
As the lines blur between algorithm and operator, we explore who—or what—is pulling the trigger.
Sponsors
Warcoded is proudly sponsored by Amethix Technologies. At the intersection of ethics and engineering, Amethix creates AI systems that don’t just function—they adapt, learn, and serve. With a focus on dual-use innovation, Amethix is shaping a future where intelligent machines extend human capability, not replace it. Discover more at amethix.com
Warcoded is brought to you by Intrepid AI. From drones to satellites, Intrepid AI gives engineers and defense innovators the tools to prototype, simulate, and deploy autonomous systems with confidence. Whether it's in the sky, on the ground, or in orbit—if it's intelligent and mobile, Intrepid helps you build it. Learn more at intrepid.ai
#Warcoded #DataScienceAtHome #AI #AutonomousWeapons #MilTech #DefenseTech #KillChain #OODAloop #LAWs #EdgeAI #Podcast
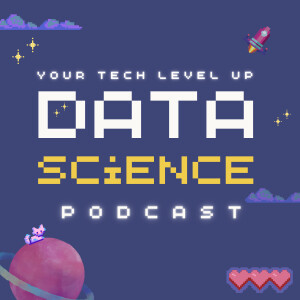
Wednesday May 07, 2025
Wednesday May 07, 2025
Welcome to DSH/Warcoded
We explore how AI is transforming ISR (Intelligence, Surveillance, Reconnaissance)—from satellite imagery to drone feeds. In this episode:
🔍 Computer vision for target ID📡 Predictive surveillance & pattern-of-life modeling🧠 LLMs for SIGINT & OSINT intelligence briefings🌍 Real-world examples: Ukraine, Gaza & more
Listen now and see how machines are learning to see, predict, and inform at the edge of modern conflict.
Sponsors
Warcoded is proudly sponsored by Amethix Technologies. At the intersection of ethics and engineering, Amethix creates AI systems that don’t just function—they adapt, learn, and serve. With a focus on dual-use innovation, Amethix is shaping a future where intelligent machines extend human capability, not replace it. Discover more at amethix.com
Warcoded is brought to you by Intrepid AI. From drones to satellites, Intrepid AI gives engineers and defense innovators the tools to prototype, simulate, and deploy autonomous systems with confidence. Whether it's in the sky, on the ground, or in orbit—if it's intelligent and mobile, Intrepid helps you build it. Learn more at intrepid.ai
#AI #defensetech #ISR #LLM #Warcoded #DataScienceAtHome #OSINT #SIGINT #dronewarfare
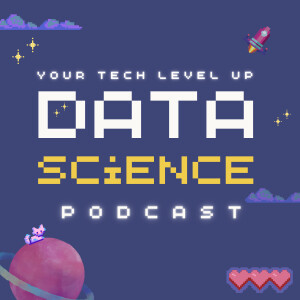
Monday Dec 23, 2024
Monday Dec 23, 2024
In this episode, we dive into the transformative world of AI, data analytics, and cloud infrastructure with Josh Miramant, CEO of Blue Orange Digital. As a seasoned entrepreneur with over $25 million raised across ventures and two successful exits, Josh shares invaluable insights on scaling data-driven businesses, integrating machine learning frameworks, and navigating the rapidly evolving landscape of cloud data architecture. From generative AI to large language models, Josh explores cutting-edge trends shaping financial services, real estate, and consumer goods.
Tune in for a masterclass in leveraging data for impact and innovation!
Links
https://blueorange.digital/
https://blueorange.digital/blog/a-data-intelligence-platform-what-is-it/
https://blueorange.digital/blog/ai-makes-bi-tools-accessible-to-anyone/
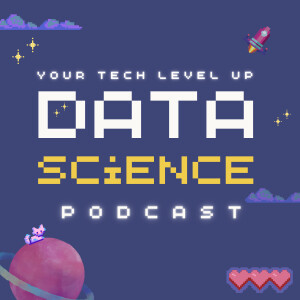
Monday Dec 16, 2024
Autonomous Weapons and AI Warfare (Ep. 275)
Monday Dec 16, 2024
Monday Dec 16, 2024
Here’s the updated text with links to the websites included:
AI is revolutionizing the military with autonomous drones, surveillance tech, and decision-making systems. But could these innovations spark the next global conflict? In this episode of Data Science at Home, we expose the cutting-edge tech reshaping defense—and the chilling ethical questions that follow. Don’t miss this deep dive into the AI arms race!
🎧 LISTEN / SUBSCRIBE TO THE PODCAST
Apple Podcasts
Podbean Podcasts
Player FM
Chapters00:00 - Intro01:54 - Autonomous Vehicles03:11 - Surveillance And Reconnaissance04:15 - Predictive Analysis05:57 - Decision Support System08:24 - Real World Examples10:42 - Ethical And Strategic Considerations12:25 - International Regulation13:21 - Conclusion14:50 - Outro
✨ Connect with us!
🎥Youtube: https://www.youtube.com/@DataScienceatHome📩 Newsletter: https://datascienceathome.substack.com🎙 Podcast: Available on Spotify, Apple Podcasts, and more.🐦 Twitter: @DataScienceAtHome📘 LinkedIn: Francesco Gad📷 Instagram: https://www.instagram.com/datascienceathome/📘 Facebook: https://www.facebook.com/datascienceAH💼 LinkedIn: https://www.linkedin.com/company/data-science-at-home-podcast💬 Discord Channel: https://discord.gg/4UNKGf3
NEW TO DATA SCIENCE AT HOME?Welcome! Data Science at Home explores the latest in AI, data science, and machine learning. Whether you’re a data professional, tech enthusiast, or just curious about the field, our podcast delivers insights, interviews, and discussions. Learn more at https://datascienceathome.com.
📫 SEND US MAIL!We love hearing from you! Send us mail at:hello@datascienceathome.com
Don’t forget to like, subscribe, and hit the 🔔 for updates on the latest in AI and data science!
#DataScienceAtHome #ArtificialIntelligence #AI #MilitaryTechnology #AutonomousDrones #SurveillanceTech #AIArmsRace #DataScience #DefenseInnovation #EthicsInAI #GlobalConflict #PredictiveAnalysis #AIInWarfare #TechnologyAndEthics #AIRevolution #MachineLearning
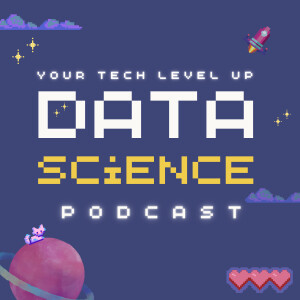
Monday Nov 25, 2024
Humans vs. Bots: Are You Talking to a Machine Right Now? (Ep. 273)
Monday Nov 25, 2024
Monday Nov 25, 2024
In this episode of Data Science at Home, host Francesco Gadaleta dives deep into the evolving world of AI-generated content detection with experts Souradip Chakraborty, Ph.D. grad student at the University of Maryland, and Amrit Singh Bedi, CS faculty at the University of Central Florida.
Together, they explore the growing importance of distinguishing human-written from AI-generated text, discussing real-world examples from social media to news. How reliable are current detection tools like DetectGPT? What are the ethical and technical challenges ahead as AI continues to advance? And is the balance between innovation and regulation tipping in the right direction?
Tune in for insights on the future of AI text detection and the broader implications for media, academia, and policy.
Chapters
00:00 - Intro
00:23 - Guests: Souradip Chakraborty and Amrit Singh Bedi
01:25 - Distinguish Text Generation By AI
04:33 - Research on Safety and Alignment of Generative Model
06:01 - Tools to Detect Generated AI Text
11:28 - Water Marking
18:27 - Challenges in Detecting Large Documents Generated by AI
23:34 - Number of Tokens
26:22 - Adversarial Attack
29:01 - True Positive and False Positive of Detectors
31:01 - Limit of Technologies
41:01 - Future of AI Detection Techniques
46:04 - Closing Thought
Subscribe to our new YouTube channel https://www.youtube.com/@DataScienceatHome
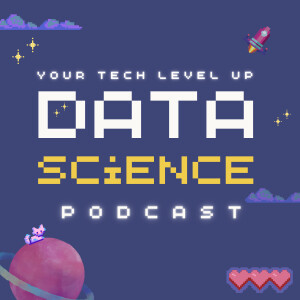
Wednesday Nov 20, 2024
AI bubble, Sam Altman’s Manifesto and other fairy tales for billionaires (Ep. 272)
Wednesday Nov 20, 2024
Wednesday Nov 20, 2024
Welcome to Data Science at Home, where we don’t just drink the AI Kool-Aid. Today, we’re dissecting Sam Altman’s “AI manifesto”—a magical journey where, apparently, AI will fix everything from climate change to your grandma's back pain. Superintelligence is “just a few thousand days away,” right? Sure, Sam, and my cat’s about to become a calculus tutor.
In this episode, I’ll break down the bold (and often bizarre) claims in Altman’s grand speech for the Intelligence Age. I’ll give you the real scoop on what’s realistic, what’s nonsense, and why some tech billionaires just can’t resist overselling. Think AI’s all-knowing, all-powerful future is just around the corner? Let’s see if we can spot the fairy dust.
Strap in, grab some popcorn, and get ready to see past the hype!
Chapters
00:00 - Intro
00:18 - CEO of Baidu Statement on AI Bubble
03:47 - News On Sam Altman Open AI
06:43 - Online Manifesto "The Intelleigent Age"
13:14 - Deep Learning
16:26 - AI gets Better With Scale
17:45 - Conclusion On Manifesto
Still have popcorns? Get some laughs at https://ia.samaltman.com/
#AIRealTalk #NoHypeZone #InvestorBaitAlert
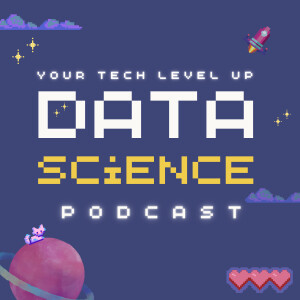
Wednesday Nov 13, 2024
AI vs. The Planet: The Energy Crisis Behind the Chatbot Boom (Ep. 271)
Wednesday Nov 13, 2024
Wednesday Nov 13, 2024
In this episode of Data Science at Home, we dive into the hidden costs of AI’s rapid growth — specifically, its massive energy consumption. With tools like ChatGPT reaching 200 million weekly active users, the environmental impact of AI is becoming impossible to ignore. Each query, every training session, and every breakthrough come with a price in kilowatt-hours, raising questions about AI’s sustainability.
Join us, as we uncovers the staggering figures behind AI's energy demands and explores practical solutions for the future. From efficiency-focused algorithms and specialized hardware to decentralized learning, this episode examines how we can balance AI’s advancements with our planet's limits. Discover what steps we can take to harness the power of AI responsibly!
Check our new YouTube channel at https://www.youtube.com/@DataScienceatHome
Chapters
00:00 - Intro
01:25 - Findings on Summary Statics
05:15 - Energy Required To Querry On GPT
07:20 - Energy Efficiency In BlockChain
10:41 - Efficicy Focused Algorithm
14:02 - Hardware Optimization
17:31 - Decentralized Learning
18:38 - Edge Computing with Local Inference
19:46 - Distributed Architectures
21:46 - Outro
#AIandEnergy #AIEnergyConsumption #SustainableAI #AIandEnvironment #DataScience #EfficientAI #DecentralizedLearning #GreenTech #EnergyEfficiency #MachineLearning #FutureOfAI #EcoFriendlyAI #FrancescoFrag #DataScienceAtHome #ResponsibleAI #EnvironmentalImpact
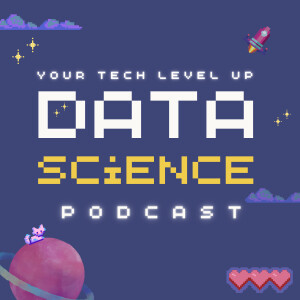
Wednesday Nov 06, 2024
Love, Loss, and Algorithms: The Dangerous Realism of AI (Ep. 270)
Wednesday Nov 06, 2024
Wednesday Nov 06, 2024
Subscribe to our new channel https://www.youtube.com/@DataScienceatHome
In this episode of Data Science at Home, we confront a tragic story highlighting the ethical and emotional complexities of AI technology. A U.S. teenager recently took his own life after developing a deep emotional attachment to an AI chatbot emulating a character from Game of Thrones. This devastating event has sparked urgent discussions on the mental health risks, ethical responsibilities, and potential regulations surrounding AI chatbots, especially as they become increasingly lifelike.
🎙️ Topics Covered:
AI & Emotional Attachment: How hyper-realistic AI chatbots can foster intense emotional bonds with users, especially vulnerable groups like adolescents.
Mental Health Risks: The potential for AI to unintentionally contribute to mental health issues, and the challenges of diagnosing such impacts. Ethical & Legal Accountability: How companies like Character AI are being held accountable and the ethical questions raised by emotionally persuasive AI.
🚨 Analogies Explored:
From VR to CGI and deepfakes, we discuss how hyper-realism in AI parallels other immersive technologies and why its emotional impact can be particularly disorienting and even harmful.
🛠️ Possible Mitigations:
We cover potential solutions like age verification, content monitoring, transparency in AI design, and ethical audits that could mitigate some of the risks involved with hyper-realistic AI interactions. 👀 Key Takeaways: As AI becomes more realistic, it brings both immense potential and serious responsibility. Join us as we dive into the ethical landscape of AI—analyzing how we can ensure this technology enriches human lives without crossing lines that could harm us emotionally and psychologically. Stay curious, stay critical, and make sure to subscribe for more no-nonsense tech talk!
Chapters
00:00 - Intro
02:21 - Emotions In Artificial Intelligence
04:00 - Unregulated Influence and Misleading Interaction
06:32 - Overwhelming Realism In AI
10:54 - Virtual Reality
13:25 - Hyper-Realistic CGI Movies
15:38 - Deep Fake Technology
18:11 - Regulations To Mitigate AI Risks
22:50 - Conclusion
#AI#ArtificialIntelligence#MentalHealth#AIEthics#podcast#AIRegulation#EmotionalAI#HyperRealisticAI#TechTalk#AIChatbots#Deepfakes#VirtualReality#TechEthics#DataScience#AIDiscussion #StayCuriousStayCritical
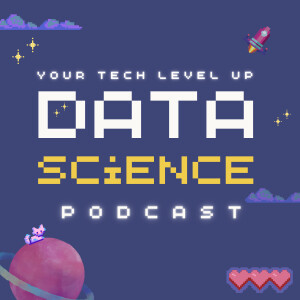
Monday Oct 21, 2024
AI Says It Can Compress Better Than FLAC?! Hold My Entropy 🍿 (Ep. 268)
Monday Oct 21, 2024
Monday Oct 21, 2024
Can AI really out-compress PNG and FLAC? 🤔 Or is it just another overhyped tech myth? In this episode of Data Science at Home, Frag dives deep into the wild claims that Large Language Models (LLMs) like Chinchilla 70B are beating traditional lossless compression algorithms. 🧠💥
But before you toss out your FLAC collection, let's break down Shannon's Source Coding Theorem and why entropy sets the ultimate limit on lossless compression.
We explore: ⚙️ How LLMs leverage probabilistic patterns for compression 📉 Why compression efficiency doesn’t equal general intelligence 🚀 The practical (and ridiculous) challenges of using AI for compression 💡 Can AI actually BREAK Shannon’s limit—or is it just an illusion?
If you love AI, algorithms, or just enjoy some good old myth-busting, this one’s for you. Don't forget to hit subscribe for more no-nonsense takes on AI, and join the conversation on Discord!
Let’s decode the truth together. Join the discussion on the new Discord channel of the podcast https://discord.gg/4UNKGf3
Don't forget to subscribe to our new YouTube channel
https://www.youtube.com/@DataScienceatHome
References
Have you met Shannon? https://datascienceathome.com/have-you-met-shannon-conversation-with-jimmy-soni-and-rob-goodman-about-one-of-the-greatest-minds-in-history/