Data Science at Home
Episodes
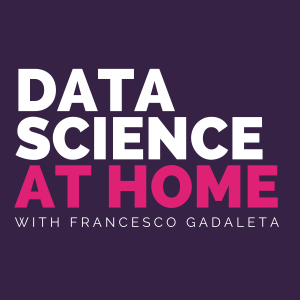
Sunday Oct 27, 2019
More powerful deep learning with transformers (Ep. 84)
Sunday Oct 27, 2019
Sunday Oct 27, 2019
Some of the most powerful NLP models like BERT and GPT-2 have one thing in common: they all use the transformer architecture. Such architecture is built on top of another important concept already known to the community: self-attention.In this episode I explain what these mechanisms are, how they work and why they are so powerful.
Don't forget to subscribe to our Newsletter or join the discussion on our Discord server
References
Attention is all you need https://arxiv.org/abs/1706.03762
The illustrated transformer https://jalammar.github.io/illustrated-transformer
Self-attention for generative models http://web.stanford.edu/class/cs224n/slides/cs224n-2019-lecture14-transformers.pdf
![[RB] Replicating GPT-2, the most dangerous NLP model (with Aaron Gokaslan) (Ep. 83)](https://pbcdn1.podbean.com/imglogo/ep-logo/pbblog1799802/data_science_at_home_podcast_cover_300x300.png)
Friday Oct 18, 2019
Friday Oct 18, 2019
Join the discussion on our Discord server
In this episode, I am with Aaron Gokaslan, computer vision researcher, AI Resident at Facebook AI Research. Aaron is the author of OpenGPT-2, a parallel NLP model to the most discussed version that OpenAI decided not to release because too accurate to be published.
We discuss about image-to-image translation, the dangers of the GPT-2 model and the future of AI. Moreover, Aaron provides some very interesting links and demos that will blow your mind!
Enjoy the show!
References
Multimodal image to image translation (not all mentioned in the podcast but recommended by Aaron)
Pix2Pix:
https://phillipi.github.io/pix2pix/
CycleGAN:
https://junyanz.github.io/CycleGAN/
GANimorph
Paper: https://arxiv.org/abs/1808.04325
Code: https://github.com/brownvc/ganimorph
UNIT:https://arxiv.org/abs/1703.00848
MUNIT:https://github.com/NVlabs/MUNIT
DRIT: https://github.com/HsinYingLee/DRIT
GPT-2 and related
Try OpenAI's GPT-2: https://talktotransformer.com/
Blogpost: https://blog.usejournal.com/opengpt-2-we-replicated-gpt-2-because-you-can-too-45e34e6d36dc
The Original Transformer Paper: https://arxiv.org/abs/1706.03762
Grover: The FakeNews generator and detector: https://rowanzellers.com/grover/
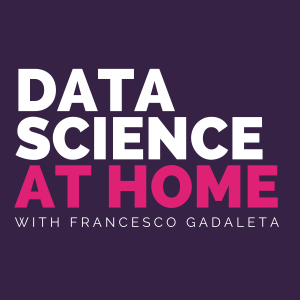
Tuesday Oct 15, 2019
What is wrong with reinforcement learning? (Ep. 82)
Tuesday Oct 15, 2019
Tuesday Oct 15, 2019
Join the discussion on our Discord server
After reinforcement learning agents doing great at playing Atari video games, Alpha Go, doing financial trading, dealing with language modeling, let me tell you the real story here.In this episode I want to shine some light on reinforcement learning (RL) and the limitations that every practitioner should consider before taking certain directions. RL seems to work so well! What is wrong with it?
Are you a listener of Data Science at Home podcast? A reader of the Amethix Blog? Or did you subscribe to the Artificial Intelligence at your fingertips newsletter? In any case let’s stay in touch! https://amethix.com/survey/
References
Emergence of Locomotion Behaviours in Rich Environments https://arxiv.org/abs/1707.02286
Rainbow: Combining Improvements in Deep Reinforcement Learning https://arxiv.org/abs/1710.02298
AlphaGo Zero: Starting from scratch https://deepmind.com/blog/article/alphago-zero-starting-scratch
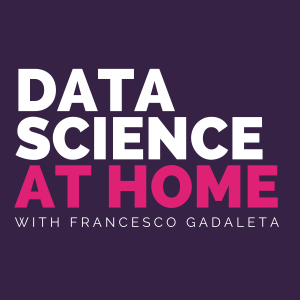
Thursday Oct 10, 2019
Thursday Oct 10, 2019
Join the discussion on our Discord server
In this episode I have an amazing conversation with Jimmy Soni and Rob Goodman, authors of “A mind at play”, a book entirely dedicated to the life and achievements of Claude Shannon. Claude Shannon does not need any introduction. But for those who need a refresh, Shannon is the inventor of the information age.
Have you heard of binary code, entropy in information theory, data compression theory (the stuff behind mp3, mpg, zip, etc.), error correcting codes (the stuff that makes your RAM work well), n-grams, block ciphers, the beta distribution, the uncertainty coefficient?
All that stuff has been invented by Claude Shannon :)
Articles:
https://medium.com/the-mission/10-000-hours-with-claude-shannon-12-lessons-on-life-and-learning-from-a-genius-e8b9297bee8f
https://medium.com/the-mission/on-claude-shannons-103rd-birthday-here-are-103-memorable-claude-shannon-quotes-maxims-and-843de4c716cf?source=your_stories_page---------------------------
http://nautil.us/issue/51/limits/how-information-got-re_invented
http://nautil.us/issue/50/emergence/claude-shannon-the-las-vegas-cheat
Claude's papers:
https://medium.com/the-mission/a-genius-explains-how-to-be-creative-claude-shannons-long-lost-1952-speech-fbbcb2ebe07f
http://www.math.harvard.edu/~ctm/home/text/others/shannon/entropy/entropy.pdf
A mind at play (book links):
http://amzn.to/2pasLMz -- Hardcover
https://amzn.to/2oCfVL0 -- Audio