Data Science at Home
Episodes
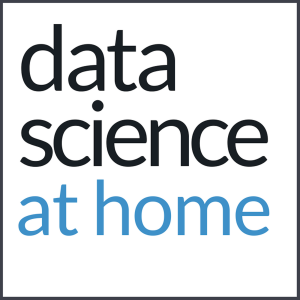
Wednesday Jan 23, 2019
Episode 53: Estimating uncertainty with neural networks
Wednesday Jan 23, 2019
Wednesday Jan 23, 2019
Have you ever wanted to get an estimate of the uncertainty of your neural network? Clearly Bayesian modelling provides a solid framework to estimate uncertainty by design. However, there are many realistic cases in which Bayesian sampling is not really an option and ensemble models can play a role.
In this episode I describe a simple yet effective way to estimate uncertainty, without changing your neural network’s architecture nor your machine learning pipeline at all.
The post with mathematical background and sample source code is published here.
![Episode 52: why do machine learning models fail? [RB]](https://pbcdn1.podbean.com/imglogo/ep-logo/pbblog1799802/logo_squared_datascience_v3_300x300.png)
Thursday Jan 17, 2019
Episode 52: why do machine learning models fail? [RB]
Thursday Jan 17, 2019
Thursday Jan 17, 2019
The success of a machine learning model depends on several factors and events. True generalization to data that the model has never seen before is more a chimera than a reality. But under specific conditions a well trained machine learning model can generalize well and perform with testing accuracy that is similar to the one performed during training.
In this episode I explain when and why machine learning models fail from training to testing datasets.
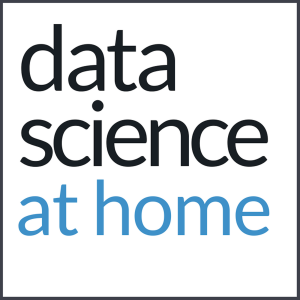
Tuesday Jan 08, 2019
Episode 51: Decentralized machine learning in the data marketplace (part 2)
Tuesday Jan 08, 2019
Tuesday Jan 08, 2019
In this episode I am completing the explanation about the integration fitchain-oceanprotocol that allows secure on-premise compute to operate in the decentralized data marketplace designed by Ocean Protocol.
As mentioned in the show, this is a picture that provides a 10000-feet view of the integration.
I hope you enjoy the show!